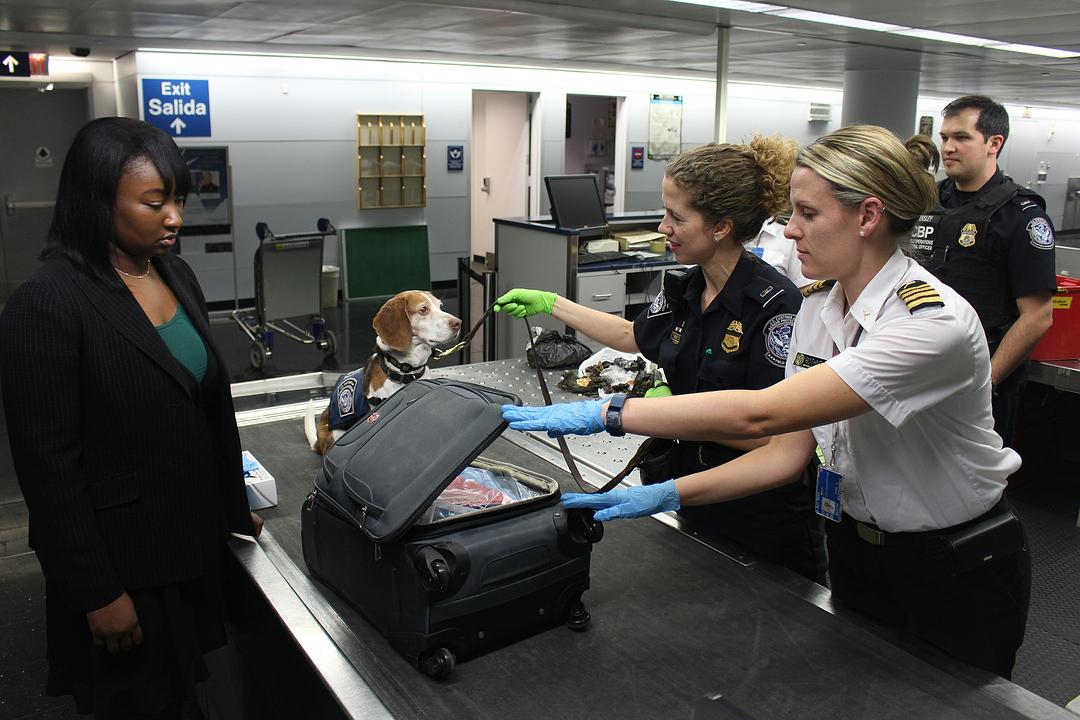
Machine Learning: A Key Player in Streamlining Operations
Machine Learning: A Key Player in Streamlining Operations
Machine learning has emerged as a transformative technology, revolutionizing the way businesses operate and compete in today's digital landscape. As companies strive to optimize their processes, reduce costs, and enhance decision-making, machine learning offers a powerful solution to streamline operations and unlock new opportunities for growth.
By harnessing the power of data and advanced algorithms, machine learning enables organizations to automate complex tasks, identify hidden patterns, and make accurate predictions—all of which contribute to improved efficiency and profitability. From supply chain optimization to customer service enhancement, the applications of machine learning in business are vast and far-reaching.
In this article, we will explore the role of machine learning in streamlining business operations, delving into its key benefits, practical applications, and best practices for successful implementation. Whether you are a business leader, an IT professional, or simply curious about the potential of this cutting-edge technology, this guide will provide valuable insights into how machine learning can transform your organization and drive sustainable growth.
Understanding Machine Learning and Its Business Impact
Machine learning (ML) is a subset of artificial intelligence that empowers computers to learn and improve from experience without being explicitly programmed. By analyzing vast amounts of data, ML algorithms can identify patterns, make predictions, and automate decision-making processes—enabling businesses to optimize operations, reduce costs, and gain a competitive edge.
The impact of machine learning on business operations is profound. By leveraging ML, organizations can:
-
Automate repetitive tasks: ML can handle mundane, time-consuming tasks such as data entry, document processing, and report generation, freeing up employees to focus on higher-value activities.
-
Enhance decision-making: ML algorithms can analyze complex datasets, uncovering hidden insights and trends that inform strategic decision-making and risk management.
-
Improve customer experiences: ML powers personalized recommendations, sentiment analysis, and chatbots, enabling businesses to deliver tailored, responsive customer service.
-
Optimize resource allocation: By predicting demand, identifying bottlenecks, and optimizing scheduling, ML helps businesses allocate resources more efficiently, reducing waste and maximizing productivity.
The potential of machine learning to streamline operations is vast, and its applications span across industries—from finance and healthcare to manufacturing and retail. As ML technologies continue to advance, businesses that embrace this transformative technology will be well-positioned to thrive in an increasingly competitive and data-driven world.
Implementing Machine Learning in Business Operations
Incorporating machine learning into business operations requires a methodical approach, beginning with the careful selection of processes that stand to benefit the most. These might include logistics management, predictive maintenance, or enhancing digital customer interactions. Each area offers distinct opportunities to leverage ML for operational improvements and cost efficiencies.
Identifying Key Business Processes
The initial step is to identify business processes where machine learning can drive significant improvements. For instance, logistics management can employ ML to optimize delivery schedules and reduce transit times, improving overall efficiency. Predictive maintenance uses ML algorithms to forecast equipment failures, enabling timely interventions and minimizing downtime. In digital customer interactions, machine learning can analyze user data to personalize experiences, increasing engagement and satisfaction.
Preparing Data for Machine Learning
Following process identification, the focus shifts to gathering and refining the data necessary for machine learning applications. Ensuring data accuracy and relevance is vital—this might involve data cleansing, integration from multiple sources, and addressing any inconsistencies. High-quality data forms the foundation of reliable ML models, which are essential for deriving actionable insights and driving business value.
Selecting Appropriate ML Algorithms and Tools
The final phase involves selecting algorithms and tools suited to the specific business needs and available data. Decisions should align with the nature of the tasks—such as using reinforcement learning for adaptive systems or clustering algorithms for customer segmentation. Platforms and tools that offer scalability and integration can support the seamless adoption of ML technologies, enhancing operational capabilities and fostering innovation within the organization.
Measuring the Impact of Machine Learning on Business Performance
Effectively leveraging machine learning in business requires clear metrics to evaluate its contributions. Establishing performance indicators that align with business objectives allows for a comprehensive assessment of machine learning implementations and their tangible benefits.
Establishing Key Performance Metrics
To accurately measure the impact of machine learning, businesses should focus on metrics that directly correlate with strategic goals. Quantifying cost reductions can provide insights into financial efficiencies achieved through automation and optimization. For operational improvements, metrics such as reductions in processing times or improvements in service delivery can highlight efficiency gains. Customer experience enhancements can be evaluated through metrics like net promoter scores or increased engagement levels, providing a holistic view of machine learning's value.
Ongoing Performance Evaluation and Refinement
Machine learning applications require continuous oversight to maintain their effectiveness. Regular evaluation of model outputs ensures they remain aligned with evolving business needs. Identifying performance gaps or areas needing refinement allows for targeted updates to algorithms or input data, fostering adaptability and sustained success. Incorporating feedback mechanisms ensures that models evolve with real-world conditions, promoting ongoing improvements and robust performance.
Demonstrating Value to Stakeholders
Conveying the impact of machine learning to stakeholders involves showcasing clear, quantifiable outcomes. Highlighting achievements such as enhanced return on investment, streamlined workflows, and improved customer interactions provides compelling evidence of success. Utilizing detailed reports and visual representations can effectively communicate the strategic advantages gained, reinforcing stakeholder confidence and supporting future machine learning initiatives.
Leveraging Machine Learning for Business Process Automation
Business process automation (BPA) evolves significantly with the integration of machine learning, enabling businesses to redefine operational efficiency. By employing sophisticated ML algorithms, businesses can streamline operations with enhanced precision, reshaping the management of routine activities. This approach facilitates the efficient handling of tasks like invoice processing and customer inquiries, allowing organizations to redirect their human resources toward more strategic initiatives.
Intelligent Automation Solutions
The fusion of machine learning with robotic process automation (RPA) results in intelligent systems that tackle complex, unstructured data effortlessly. In sectors such as logistics and retail, these systems can automatically classify and process varied data inputs, minimizing manual intervention and reducing processing time. For instance, ML-enhanced RPA can optimize supply chain logistics by dynamically adjusting routes based on real-time data, leading to substantial cost savings. This integration amplifies operational scalability and delivers greater flexibility in responding to market changes.
Optimization and Continuous Monitoring
To maximize the potential of machine learning-driven automation, continuous oversight and refinement are critical. Implementing robust feedback mechanisms allows businesses to adapt systems to evolving data patterns and operational demands effectively. By regularly evaluating automated workflows, organizations can swiftly identify and rectify inefficiencies, ensuring sustained performance improvements. This ongoing process not only bolsters system reliability but also empowers businesses to maintain a competitive edge through agile and responsive operations.
Enhancing Decision-Making with Machine Learning Insights
Machine learning empowers organizations to refine their decision-making processes by transforming extensive datasets into valuable insights. Advanced algorithms sift through diverse data sources, uncovering intricate relationships and patterns that might remain unnoticed through traditional analysis. This capability allows businesses to anticipate market dynamics, customer preferences, and operational challenges, thus enabling more precise strategic planning.
Dynamic Analytics for Informed Strategy
Integrating dynamic analytics into organizational frameworks equips businesses with the capability to swiftly adapt to new developments and seize emerging opportunities. Machine learning models process and interpret data in real time, providing decision-makers with timely insights that are crucial for proactive strategy adjustments. This continuous flow of information ensures that businesses remain aligned with their strategic goals while mitigating potential risks with greater precision, fostering a nimble and responsive decision-making environment.
Balancing Algorithmic Insights with Human Judgment
While machine learning excels in data processing, the synthesis of algorithmic insights with human expertise is essential for contextually nuanced decision-making. Human intuition and strategic acumen add depth to the interpretation of data-driven insights, ensuring that decisions reflect both analytical rigor and experiential understanding. By marrying these elements, businesses can formulate strategies that leverage the predictive accuracy of machine learning while incorporating the critical judgment and creativity of human experts, driving innovation and sustained competitive advantage.
Future-Proofing Your Business with Machine Learning
In an ever-evolving technological landscape, businesses must remain vigilant and adaptive to leverage the latest in machine learning technology. Actively engaging with recent developments and exploring new applications ensures that your organization can integrate advanced ML solutions to sustain its competitive edge. By embracing innovative tools and methodologies, businesses can seamlessly align with industry shifts and anticipate market changes, staying ahead of disruptions and capitalizing on emerging opportunities.
Cultivating a dynamic environment of continuous growth and exploration is essential for harnessing the full potential of machine learning. Encouraging teams to pursue creative applications of ML fosters a workplace where innovation thrives. By providing access to specialized training, cutting-edge resources, and experimental projects, organizations empower their workforce to develop novel solutions that address evolving business challenges. This commitment to growth and adaptation enhances the organization's resilience and flexibility, enabling it to navigate the complexities of a rapidly changing market.
Strategic collaboration with external experts, academic leaders, and industry innovators amplifies an organization's machine learning capabilities. By engaging with a network of external stakeholders, companies can gain valuable insights and share best practices that enrich their ML strategies. These partnerships can lead to joint ventures, collaborative research, and shared technological advancements, driving the organization's ability to implement cutting-edge solutions. Through these strategic alliances, businesses ensure they remain at the forefront of technological innovation, securing their position as leaders in their respective industries.
Ethical Considerations and Responsible AI Practices
As machine learning becomes more integral to business operations, addressing ethical considerations is paramount. Establishing a solid ethical framework for ML deployment is essential to ensure that AI solutions align with societal values and promote trust among stakeholders. These frameworks should focus on key areas such as impartiality, clarity, and accountability, which are critical for maintaining ethical integrity and avoiding potential pitfalls associated with AI misuse.
Implementing Robust Ethical Frameworks
Creating comprehensive ethical guidelines involves a proactive approach to identifying and mitigating biases inherent in data and algorithms. By instituting clear protocols, businesses can ensure that machine learning systems operate equitably and do not perpetuate existing societal inequalities. Clarity in machine learning processes is vital—it enables stakeholders to comprehend decision-making pathways and fosters accountability. By defining responsibilities and establishing monitoring mechanisms, organizations can maintain ethical standards while achieving technological advancements.
Conducting Ongoing Fairness Assessments
Regular assessments of machine learning models are crucial to uphold fairness and prevent discriminatory outcomes. These evaluations involve a thorough analysis of data inputs, algorithmic processes, and outputs to identify and address biases, ensuring equitable treatment across diverse demographic groups. By implementing these checks, businesses can safeguard public trust and demonstrate a commitment to ethical AI practices. Maintaining a dynamic review process allows organizations to adapt to evolving societal norms and regulatory requirements, reinforcing their dedication to responsible AI usage.
Emphasizing Model Interpretability
The interpretability of AI models is vital for demystifying the complex decision-making processes of machine learning systems. By providing clear insights into how models arrive at specific conclusions, businesses empower stakeholders and regulators to scrutinize and validate AI-driven decisions. This transparency not only enhances human oversight but also facilitates necessary interventions when models deviate from ethical norms. By prioritizing interpretability, organizations ensure that their machine learning initiatives remain accountable and aligned with broader societal expectations, fostering an environment of trust and collaboration.
As machine learning continues to revolutionize business operations, it is evident that embracing this technology is no longer a choice but a necessity for organizations seeking to thrive in the digital age. By harnessing the power of machine learning, you can streamline processes, enhance decision-making, and unlock new opportunities for growth. If you're ready to embark on your AI-driven transformation journey, Contact Dwight Davis for AI and digital strategy consulting services—we're here to help you navigate the complexities of machine learning and build a future-proof business.